AI and the MD: Doctors will soon use artificial intelligence to help diagnose and treat patients, opening up new possibilities for better health
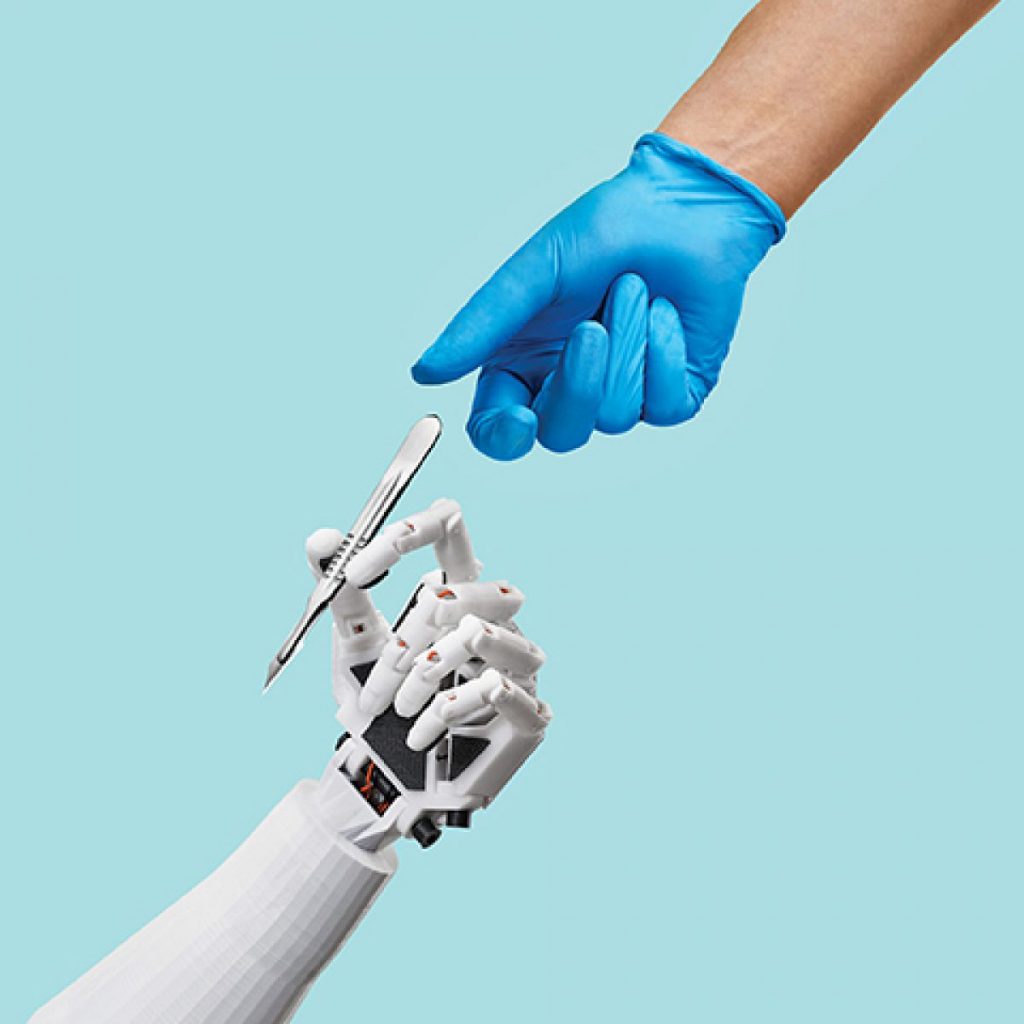
If you’ve never heard of gene TP53, consider yourself lucky. It’s a “tumour suppressor gene.” It generates a protein that keeps your cells from growing and dividing too quickly.
Many people only get to know this gene if they have a rare mutation that stops it from working properly – a condition called “Li-Fraumeni Syndrome.” People with the syndrome will almost certainly develop cancer. If they survive one type of cancer, they’ll likely get another. And another. And another.
“It’s not a question of if. It’s a question of when,” says Anna Goldenberg, a professor of computer science at U of T and a senior scientist at the Hospital for Sick Children. In one of her research projects, she’s using machine-learning algorithms to try to answer “the question of when” for families who have a child with Li-Fraumeni Syndrome.
Some children will get cancer in their very early years. Others can be tumour-free for decades. If Goldenberg’s algorithms can predict near-term risks, it could save some families years of blood tests, CT scans and other invasive monitoring that young children often can’t understand.
“It’s very hard to get a kid to lie still for a scan when they are two or three. It’s impossible to explain to them why they need this to live,” Goldenberg says. “It becomes very burdensome on their families.”
Goldenberg, who is also a member of the health subgroup at Toronto’s Vector Institute for Artificial Intelligence, is working on an algorithm that could use markers in a single blood sample to predict whether a baby with Li-Fraumeni Syndrome will develop cancer before the age of six. Those not at immediate risk can be spared a highly medicalized life for their first few years.
Her project, being conducted at Sick Kids, could help doctors and patients reduce their reliance on guesswork, subjective opinions and one-size-fits-all medical solutions. Machine-learning algorithms could help with almost every type of health problem, from cancer to diabetes to depression – and improve risk assessment, diagnosis, prevention, management and treatment.
Machine learning, a branch of artificial intelligence research, involves training computers (or having them train themselves) on large data sets. The computers learn to identify patterns and make predictions about the world based on this training. Machine learning has shaken up fields ranging from finance to voice recognition to airport security.
For health-related machine-learning applications, Ontario presents an especially promising training ground. In addition to being home to a deep pool of AI talent, the province has invested in developing electronic medical records. Because of Canada’s universal health-care system, these records could provide (if patient privacy can be protected) valuable source data, covering rich and poor people, urban and rural residents, men and women, not to mention a wide range of ethnicities.
Hidden in that vast, complex mass of data are answers to many questions that once seemed impenetrable. The human mind might not be able to winnow out the kinds of solutions Goldenberg seeks, but machines offer hope.
Traditional medical studies almost always require simplification, removing as many variables as possible to allow researchers to draw conclusions from the data. But that means their solutions are often incomplete or don’t work for everyone.
For instance, asthma medicines tend to be tested on a tiny portion of the general asthmatic population. For simplicity’s sake, most clinical trials only use asthma sufferers who don’t have any complications – which eliminates more than 90 per cent of people with asthma. This means a medication approved for general use might be wrong for most asthma sufferers.
“It’s not because doctors are evil or malicious,” says Marzyeh Ghassemi, who will join the Vector Institute this summer and start at U of T in the fall as a professor in the department of computer science. “It’s because randomized controlled trials are expensive to run and it’s challenging to get reasonable statistical guarantees about a drug’s performance.”
People with multiple conditions make research data complicated, but artificial intelligence can help sort out just this kind of difficulty. Complexity becomes an asset rather than a problem for AIs, which use it to increase knowledge about how drugs and other treatments work in different, specific scenarios.
Several Vector researchers are concentrating their attention on chronic conditions. Ghassemi, for example, is working on new apps to improve how people manage diagnosed depression. Even if a person with depression has regular visits with medical professionals, “most of people’s lives are not spent in doctors’ offices,” she says. This means that warning signs, destructive behaviour and downward spirals can go undetected for too long.
But in an age of smartphones, smart watches and other wearable technologies, people can use their devices as background monitors or “passive sensors.” The user does not have to actively log or enter data themselves – an app on a wearable device could track both biological information such as heart rate and sleep cycles, and also social behaviours like how often you leave your house or contact your friends and family. It could analyze changes to your speech patterns and the tone of your voice. Changes to any of these things could be warning signs that an individual is descending into depression.
“It makes a lot of sense to look at passive sensing data to try and understand if there are some characteristics of behaviour, both at the population level and also at an individual level, that affect the way you feel and the way you deal with your chronic condition,” Ghassemi says.
An AI-driven app could analyze an ongoing stream of data and flag issues that are highly specific to the wearer. The guidance it offers would be adapted to each individual.
“Maybe what’s going to indicate a need to modify medication or intervene for you is that you haven’t been outside for a long time. And maybe for me, it’s that I haven’t called my mother in a long time,” she says. Such an app could prompt users to take action, such as going for a walk or talking to a friend.
Not only can machine-learning algorithms analyze huge amounts of data, they can find connections across many kinds of data sets: biological, genetic, demographic, behavioural, socioeconomic and so on. When applied properly, this can help doctors and medical researchers understand better what’s going on with the health of both large populations and individual patients.
While Ghassemi’s project focuses on depression (and so far is looking only at a user’s location data), the same tools could be adapted for managing other chronic diseases such as diabetes or Chronic Obstructive Pulmonary Disease (COPD).
Currently, many cancers don’t fit into the category of chronic conditions. But Quaid Morris, a U of T professor in molecular genetics and a Vector faculty member, hopes to expand that list by using AI to improve our knowledge of tumours. “The goal is to transform more cancer types into chronic diseases,” Morris says.
The condition wouldn’t go away, but with treatment, it wouldn’t shorten your life very much or significantly diminish its quality.
He’s using machine-learning algorithms to combine molecular data with clinical information and learn more about how tumours evolve. It’s a relatively new research area, which means many of the questions he’s trying to answer are basic science. But it’s basic science with a clear goal for applying that knowledge.
Why do some people get cancer and others don’t? Why would a particular chemotherapy cocktail work for one person and not another? How do we know who’s at risk for which cancers? How can we give each individual the most effective treatment for their unique circumstances? The answers to these questions involve a complex mix of factors that are far easier to address with the assistance of machines.
For example, between five and 10 per cent of breast cancers are thought to be caused by specific hereditary genetic mutations. But not everybody with one of these mutations gets breast cancer.
Other genetic variations can dampen (or heighten) the effects of “breast cancer genes.” Diet, lifestyle, other health issues, pregnancy, body chemistry and a huge range of other factors might also affect who gets cancer and who doesn’t. All of these potential causes interact with each other in a way that is opaque to even the best human minds.
Machine-learning algorithms offer the possibility of winnowing out the information hidden within the messy network of (potentially) thousands of interrelated variables. They can detect patterns, find correlations and identify causes and risk factors that could ultimately explain why, among women with the mutation, one might benefit from pre-emptive mastectomy, while another might never need any treatment at all.
The algorithms might discover a pattern of gene interactions that shields a certain subpopulation from developing cancer. Or find that people who undergo chemotherapy become more prone to certain cancers. “Your model can be very complex in ways that it couldn’t be before, because either you would have trouble getting enough data, or the computation would take too long to get insights,” Morris says. “Those two things have improved.” Algorithmic analysis will offer patients and doctors better information about the optimal course of cancer treatment.
In addition to pursuing their specific research projects, the members of the Vector Institute health subgroup are also interested in the broad impact of their work and the direction of AI-related health research in general. They all agree that in the near future, AI’s role will be to augment the capacity of human medical professionals, not to replace them.
“You need humans for the subjective things,” says Morris. “Human brains are good at decision-making – using imperfect information and a wealth of experience to make complex choices. It’s very hard to get an artificial intelligence to make those kinds of intuitive decisions or judgment calls.”
Smart algorithms will continue to expand their presence in hospitals and doctors’ offices, as well as on our personal devices. They will change how we approach disease. Doctors, nurses and patients can all expect to have better data and analysis to help them make smarter, better informed medical decisions.